Yutai Zhou
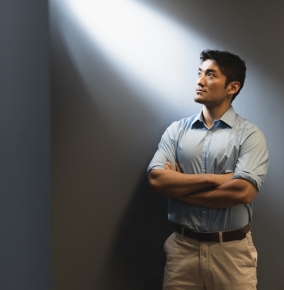
Where did you grow up?
I was born and raised in Shanghai, which is often described as the New York City of China. I spent roughly the first half of my life there, before moving to the suburbs of West Palm Beach, a comparatively smaller city in South Florida.
What do you like about working at the Laboratory?
I have a broad range of technical interests, and prefer to work on multiple projects. The Laboratory is an interdisciplinary place where experts from many different fields come together to solve important problems. Given the wide range of opportunities and resources here, it is impossible to run out of cool projects to tackle!
What aspects of your work do you take the most pride in?
Due to my interests and experiences in the field of reinforcement learning, I have been given the unique opportunity to help steer the research direction of one of the Laboratory's RL research projects, MeRLin (which stands for Mission-ready Reinforcement Learning). As an early-career researcher, the level of autonomy given to me is simultaneously daunting yet rewarding.
What is reinforcement learning? What makes it both an exciting and challenging area of research?
Reinforcement learning (RL) is one of the three dominant machine learning paradigms, next to supervised and unsupervised learning. It has its roots in optimal control and psychology, and can be best described as trial-and-error learning. I work in multi-agent RL, which is a variant that is concerned with multiple learning agents in an environment, interacting with various dynamics: competitive, cooperative, mixed, etc. The presence of other learning agents introduces extra difficulties to the single-agent RL problem, as the quality of the agents’ everchanging behavior will affect the state of the environment for every agent involved.
Intelligence is anything that is able to adjust its behavior in light of new knowledge; this definition applies to both computer agents and AI. There is no denying that AI will be an integral part of the future; as a result, our future will surely involve complicated many-agent interactions consisting of both human and computer agents. Teaching computer agents to communicate, cooperate, and collaborate with humans and with other agents is a crucial challenge to building a safer future. Multi-agent RL provides a great lens to study the problem.
What do you like to do outside of work?
My biggest hobby outside of work is powerlifting, which is a strength sport that has introduced me to many great friends. I can also frequently be found hiking at the Middlesex Fells Reservation, or enjoying a book or anime episode at any tea shop that has great matcha, oolong, or lavender tea.