Supercomputing can help cyber analysts find suspicious behavior on the internet
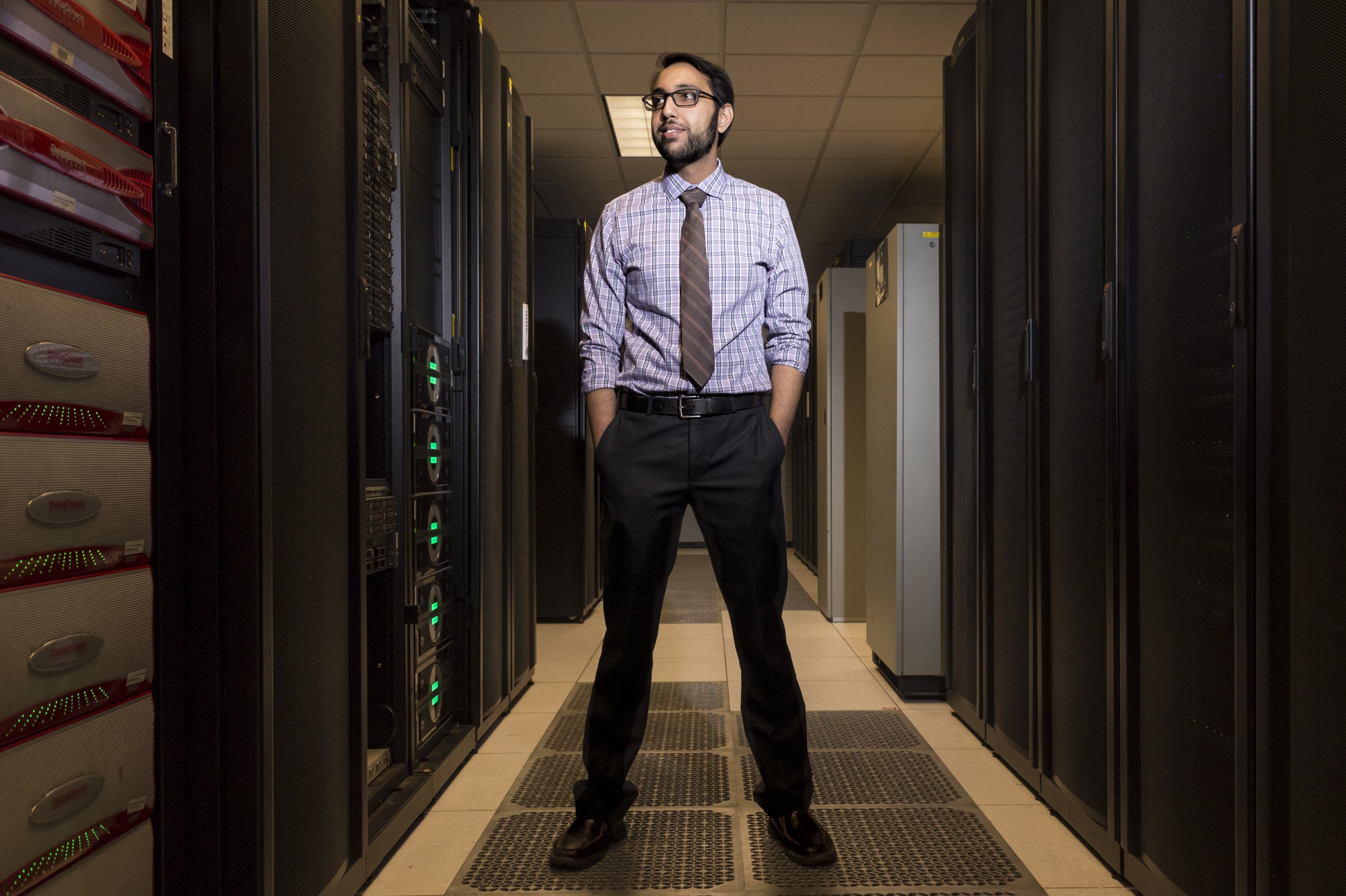
Identifying cybersecurity threats from raw internet data can be like locating a needle in a haystack. The amount of internet traffic data that is generated in a 48-hour period, for example, is too massive for one or even 100 laptops to process into something digestible for human analysts. Because of this issue, analysts rely on sampling to search for potential threats — they select small segments of data to look at in depth, hoping to find suspicious behavior. While this type of sampling may work for some tasks, such as identifying popular IP addresses, it is inadequate for finding subtler threatening trends.
"If you're trying to detect anomalous behavior, by definition that behavior is rare and unlikely," said Vijay Gadepally, a senior staff member at the Lincoln Laboratory Supercomputing Center (LLSC). "If you're sampling, it makes an already rare thing nearly impossible to find."
Gadepally is part of a research team at the Laboratory that believes supercomputing can offer a better method for identifying these subtle trends — one that grants analysts access to all pertinent data at once. In a recently published paper, the team successfully condensed 96 hours of raw, 1-gigabit network link internet traffic data into a query-ready bundle. They compressed these data by running 30,000 cores of processing (equal to about 1,000 laptops) at the LLSC located in Holyoke, Massachusetts. The data are stored in the MIT SuperCloud, where it can be accessed by anyone with an account.
"[Our research] showed that we could leverage supercomputing resources to bring in a massive quantity of data and put it in a position where a cybersecurity researcher can make use of it," Gadepally explained.
An example of the type of threatening activity that requires analysts to dig in to such a massive amount of data is that from command-and-control (C&C) servers. These servers issue commands to devices infected with malware in order to steal or manipulate data. Gadepally likens their pattern of behavior to that of spam phone callers — while a normal caller might make and receive an equal number of calls, a spammer would make millions more calls than they receive. It's the same idea for a C&C server, and this pattern can be found only by looking at much more data over a long period of time.
"The current industry standard is to use small windows of data, where you toss out 99.99 percent," Gadepally said. "We were able to keep 100 percent of the data for this analysis."
The team plans to spread the word about their ability to compress such a large quantity of data. They hope that analysts will take advantage of this resource to take the next step in cracking down on threats that have so far been elusive. They are also working on ways to better understand what "normal" internet behavior looks like as a whole, so that threats can be more easily identified.
"Detecting cyber threats can be greatly enhanced by having an accurate model of normal background network traffic," said Jeremy Kepner, a Laboratory Fellow at the LLSC who is spearheading this new research. Analysts could compare the internet traffic data they are investigating with these models to bring anomalous behavior to the surface more readily. "Using our processing pipeline, we are able to develop new techniques for computing these background models."
As government, business, and personal users increasingly rely on the internet for their daily operations, maintaining cybersecurity will remain an essential task for researchers. Supercomputing is an untapped resource that can help them in this endeavor.