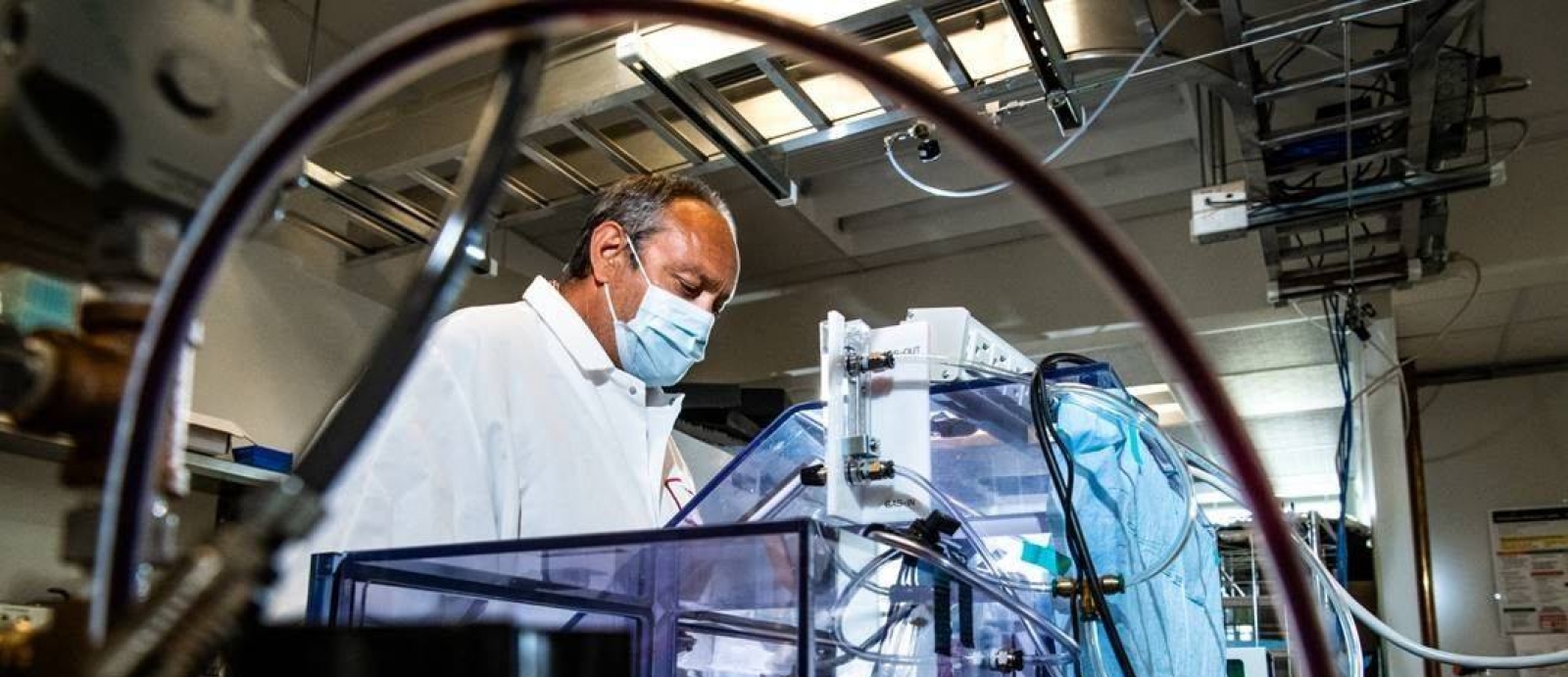
A pandemic demands novel technology
How to keep healthcare workers safe, how to monitor and inhibit the spread of infection, and how to predict the progression of the outbreak were among the most pressing questions. Below is a sampling of technologies that Lincoln Laboratory has brought to the national and global response to COVID-19.
Tracking Infections
Soon after PACT's development, Apple and Google integrated a similar framework into their jointly created Exposure Notification System. The system has been enabled on millions of smartphones worldwide and has resulted in hundreds of thousands of exposure notifications. It has also been integrated into official contact tracing efforts in several states, including Pennsylvania, California, Virginia, New York, and New Jersey, and in Washington D.C.
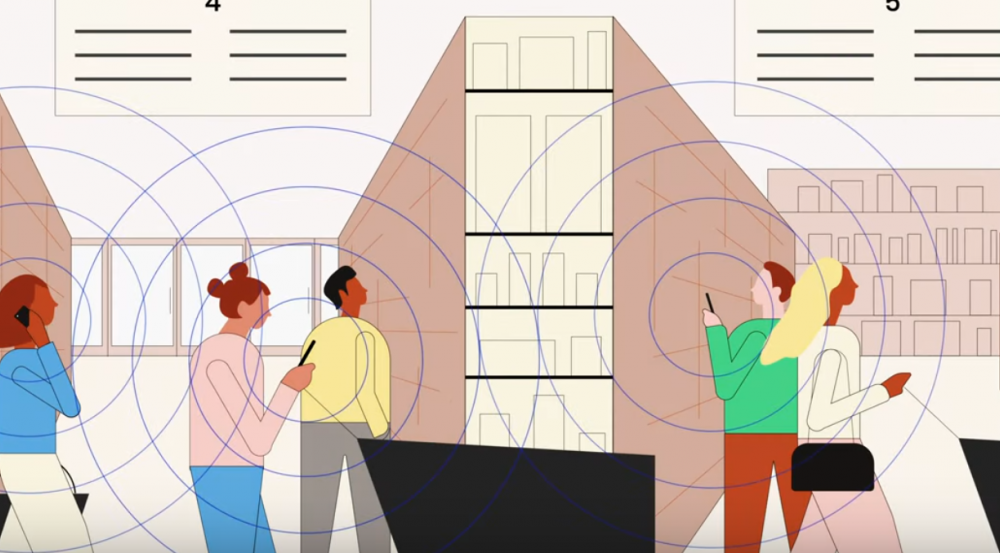
Closer to home, MIT leadership considered its own abilities to track infections and control outbreaks as students returned to campus in the fall. The MIT Quest for Intelligence and Lincoln Laboratory developed a situational awareness platform, called the MIT COVID-19 Response System, that models population densities in campus buildings, predicts the flow of people at entrance points, and identifies potential hotspots and infection risks. The system has helped MIT leaders make daily decisions regarding the health and status of the MIT community.
Readying PPE
In early spring, the Laboratory conducted a study to estimate daily demand for personal protective equipment (PPE) for frontline workers in Massachusetts. The analysis was conducted for the Massachusetts Manufacturing Emergency Response Team (M-ERT) to guide local businesses’ production of PPE. To estimate totals, the team modeled the in-hospital demand that scales with the number of hospitalized COVID-19 patients, and the in- and out-of-hospital demand that must be sustained over the outbreak duration. They estimated a daily demand through November 2021 of approximately 300,000 N95 masks, two million glove pairs, one million surgical masks, and 100,000 surgical gowns. Carolyn Kirk, M-ERT executive director, hailed the Laboratory’s analysis as providing a “vital public service.”
The Laboratory also tested the quality of various foreign-sourced N95 respirator masks to help frontline workers avoid the use of faulty or fraudulent materials. The tests, which measured filtration efficiency and liquid penetration, were conducted on hundreds of masks submitted by hospitals, first responders, and local governments, and the results were routinely published to the Massachusetts Department of Public Health’s website.
Predicting Outbreaks
As the federal response to the COVID-19 pandemic began to ramp up in early March, the U.S. Northern Command (USNORTHCOM) was deployed to provide medical staff to hospitals, construct healthcare facilities, and support logistics. However, the command soon found that they were deploying to locations that did not need their help. They reached out to the Laboratory requesting a capability to predict where an outbreak would occur and what resources would be needed there. Within 24 hours, a team began building analytics showing outbreak predictors and healthcare capacity shortfalls in every county across the United States. These predictive analytics were delivered daily to the combatant commander, who used the data to reconfigure forces and proactively deploy them to locations in advance of regional outbreaks. The team also developed novel analytics to guide USNORTHCOM in the event of a multi-disaster environment, such as responding to hurricanes in a COVID-19-positive environment.
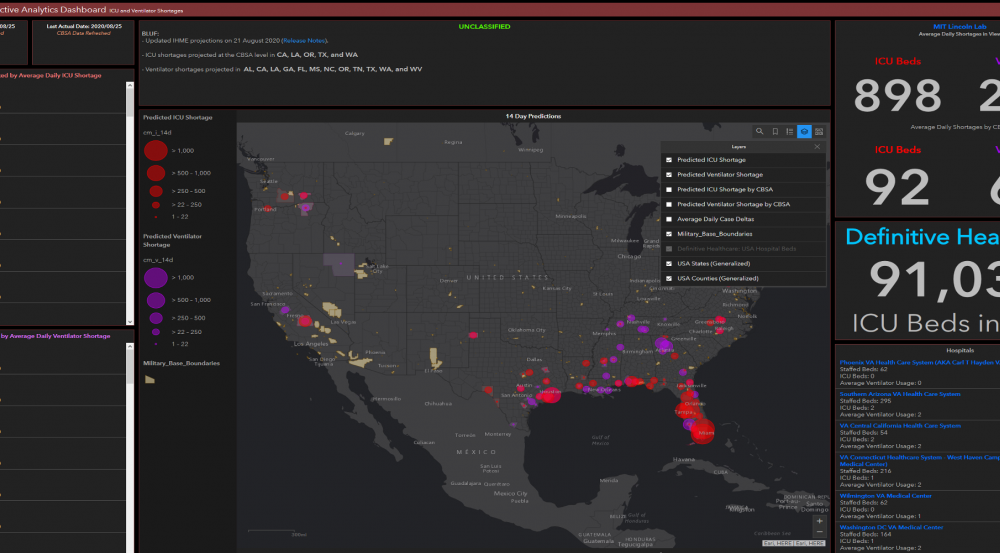
Predicting and monitoring COVID-19 infections within the armed services has also been a national security priority. Today, wearable sensors can monitor service members’ health to provide early warning of injury. In the era of the COVID-19 pandemic, wearable sensors are being investigated to determine if physiological status monitoring can provide early warning of SARS-CoV-2 exposure and COVID-19. A large number of ongoing studies funded by U.S. government agencies seek to quantify the use of wearables for an integrated early-warning capability. These studies are using a broad set of wearable devices, but share a common goal of developing artificial intelligence (AI) models for early warning of infection. These datasets represent an unprecedented collection of physiological data that can be used to answer many key questions beyond whether an individual is infected.
In collaboration with and under funding from the Defense Threat Reduction Agency, Biomedical Advanced Research and Development Authority, U.S. Army Medical Materiel Development Activity, and National Institutes of Health, Lincoln Laboratory has been building a platform to store and curate data and AI models from many of these ongoing studies. This platform is supporting the rapid development and validation of algorithms not just for providing early warning of COVID-19 infection, but also for detecting disease progression and recovery, and potentially for characterizing diseases and pathogens.
Mitigating Transmission
The aerosol hazards caused by COVID-19 respiratory therapies were identified as a key information gap in understanding virus transmission. To help quantify these hazards, the Laboratory collaborated with Tufts Medical Center to collect and analyze aerosol data. Air samples were collected during treatment of COVID-19 patients at Tufts Medical Center and analyzed for the presence of SARS-CoV-2 RNA at Tufts University. Aerosol measurements were initially collected from healthy participants undergoing these respiratory treatments to help guide sampling strategies. The team determined the spatial and size distribution of viral aerosols within a patient’s room and characterized the infectivity of the aerosols. The research has helped clinicians evaluate risk during treatment and prioritize PPE usage.
The Laboratory is also working with the Department of Homeland Security Science and Technology Directorate to better understand virus transmission in public transit and to provide transit authorities with practical options for mitigating its spread. In a series of experiments, the researchers disseminated safe viral simulants in various droplet sizes to mimic an infected passenger coughing or sneezing on buses and subway cars (occupied only by research staff). The particles are coated with DNA “barcodes” to allow the researchers to detect and track them in the air, on surfaces, and on clothing. Through these experiments, the team is assessing the effectiveness of mitigation strategies, such as using different HVAC settings, opening windows and doors, and limiting occupancy levels. These tests are being conducted in partnership with New York City’s Metropolitan Transportation Authority and are expected to be applicable to other transit systems.
Countering Disease
When a new pathogen emerges, identifying and validating medical countermeasures (MCMs)—drugs to treat a disease—can take months to years. In response to the COVID-19 outbreak, the Laboratory piloted a system under development with the Defense Threat Reduction Agency to speed MCM development, with a focus on restoring, sustaining and protecting warfighter health and performance. The goal of the program is to develop a systematic approach to rapidly identify, assess, and recommend MCMs to any new pathogen by focusing on screening MCM candidates that have already been FDA approved as treatments for other conditions.
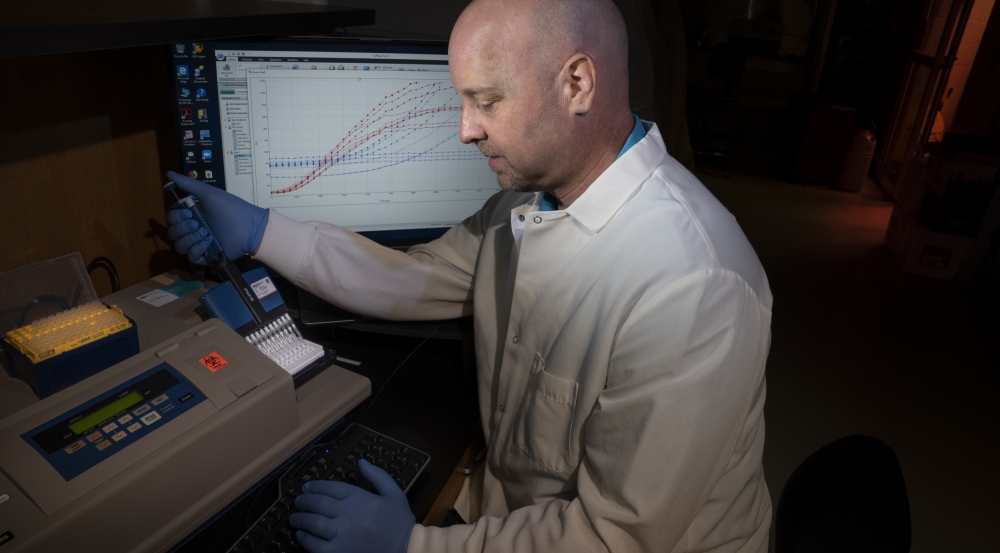
Using the SARS-CoV-2 pathogen as a test case, the team prototyped a drug-candidate review pipeline and developed recommendations for actions to accelerate timelines. As part of this work, Laboratory researchers leveraged advanced DNA sequencing to generate large biological datasets. These datasets were used to develop a machine learning framework to answer biologically relevant questions about the pathogen and identify evidence to support inclusion or exclusion of candidate drugs for human studies. Candidate drugs were then analyzed to determine uncertainties, such as dosage, combinations with other drugs, or regulatory restrictions. In a related effort, the Laboratory applied a novel method for rapid, reproducible, and high-throughput screening of key molecular interactions to rapidly assess potential MCMs for efficacy in treating SARS-CoV-2 infections.
Because significant disease outbreaks are unpredictable, having an analysis infrastructure in place to rapidly identify MCMs is vital. The lessons learned from this project and the MCM identification system architectures will enable a more rapid and robust response to future pandemics while providing timely recommendations to address the current COVID-19 pandemic.