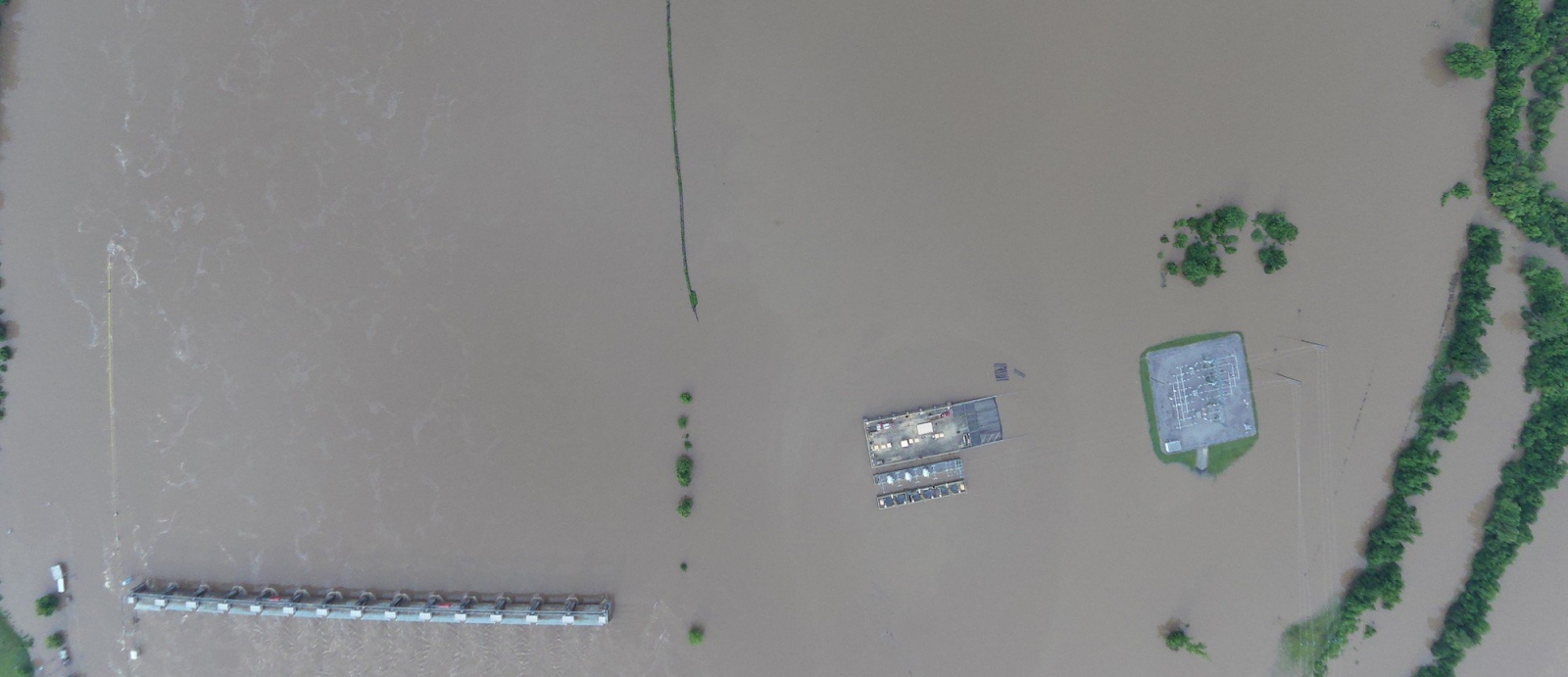
AI thinks this flood photo is a toilet. Fixing that could improve disaster response.
Andrew Weinert and his colleagues were deeply frustrated. After Hurricane Maria struck Puerto Rico, the researchers from MIT's Lincoln Laboratory were hard at work trying to help the Federal Emergency Management Agency (FEMA) assess the damage. In hand they had the perfect data set: 80,000 aerial shots of the region taken by the Civil Air Patrol right after the disaster.
But there was an issue: there were too many images to sort through manually, and commercial image recognition systems were failing to identify anything meaningful. In one particularly egregious example, ImageNet, the golden standard for image classification, recommended labeling an image of a major flooding zone as a toilet.
"There was this amazing information content, but it wasn’t accessible," says Weinert.
They soon realized this problem isn't unique. In any large-scale disaster scenario, teams of emergency responders like FEMA could save significant time and resources by reviewing details of on-the-ground conditions before their arrival. But most computer vision systems are trained on regular day-to-day imagery, so they can't reliably pick out relevant details in disaster zones.
The realization compelled the team to compile and annotate a new set of photos and footage specific to emergency response scenarios. They released the data set along with a paper this week in the hopes that it will be used to train computer vision systems in the future.