Interpretable Neural Networks for Visual Reasoning
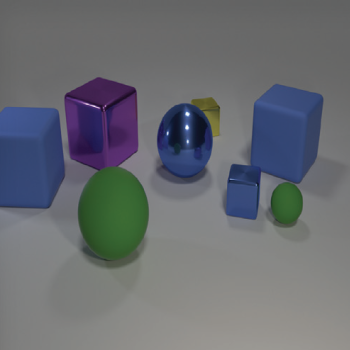
Researchers at Lincoln Laboratory have developed a neural network model called Transparency by Design that not only answers complex questions about the contents of images but also makes its reasoning understandable to humans. The network explicitly models each step in its process for deriving the answer and "shows its thinking" as it solves the query.
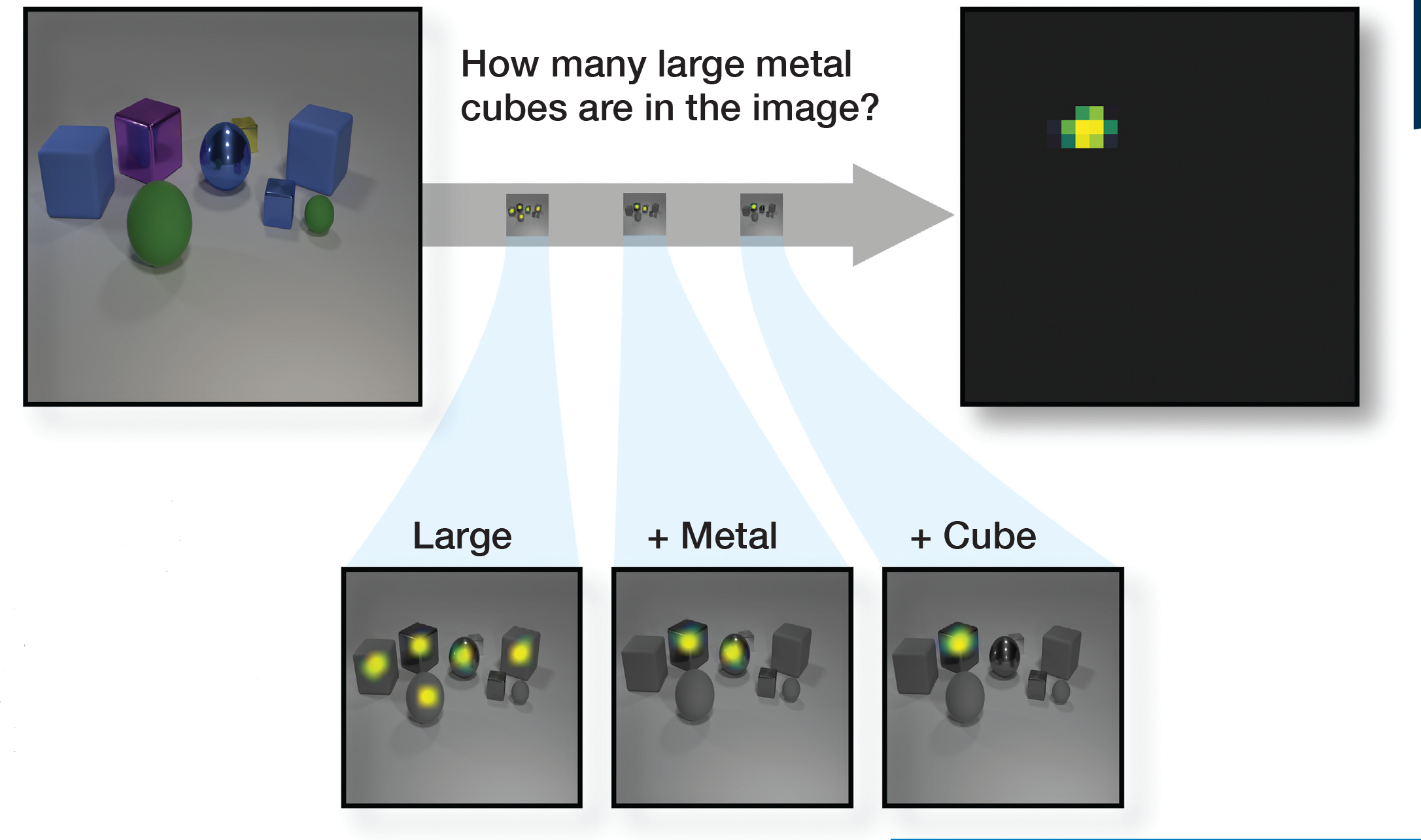
The model is provided with the image (top left) and a question (top middle). It parses the question to determine the series of operations needed to answer the question and composes these operations (right) to arrive at the answer (top right). Each of these steps is accessible to a user and can be understood and verified.
Because the neural network outputs the regions of the image that the model finds important at each step, it allows a user to build intuition about how the model works and to double-check its performance. Transparency by Design's process leads to diagnostic insights; when the model returns an incorrect answer, the visibility of its "thought process" allows the user to understand what led to the faulty answer. These capabilities that provide interpretability also provide users a level confidence in the model's accuracy.
Transparency by Design's modularity allows analysts to reuse atomic operations (e.g., find red objects, find cubes, compare colors, etc.) and naturally enables the breakdown of questions into their constituent parts and the composition of operations for expressivity.
Transparency by Design achieved state-of-the-art performance on an academic dataset, surpassing similar modular approaches. It also achieves state-of-the-art results on a challenging generalization task.
Benefits
- Modular neural network breaks questions down into their logical components
- Intermediate model outputs allow human operators to understand network operation
- State-of-the-art performance on challenging academic datasets
Potential Use Cases
- Software development
- Artificial intelligence applications
Additional Resources
This software is available
https://github.com/davidmascharka/tbd-nets
D. Mascharka, P. Tran, R. Soklaski, and A. Majumdar, “Transparency by Design: Closing the Gap Between Performance and Interpretability in Visual Reasoning,” paper in Proceedings of the 2018 IEEE/CVF Conference on Computer Vision and Pattern Recognition, 2018, pp. 4942–4950.