Graph Exploitation Symposium emphasizes responsible artificial intelligence
Lincoln Laboratory’s Graph Exploitation Symposium (GraphEx) brought together academic, government, and industry researchers to explore the state of the art in network science. Network science studies the relationships within complex systems, and its insights are applied in fields as disparate as chemistry, business, medicine, and social media.
This year’s two-day virtual event, held from May 16 to 17, featured 14 presentations in addition to online poster sessions. Several sessions of the symposium emphasized themes of ethical, responsible artificial intelligence (AI) design and use.
“Recognizing the important conversations happening in the broader community about responsible AI and designing algorithms that are ethical, fair, and resilient to corruptions, we wanted to bring these themes to our symposium as they relate to studying networks and graph-based data,” said GraphEx co-chair Rajmonda Caceres, a senior researcher in the Laboratory’s Integrated Missile Defense Technology Group.
Ensuring responsible AI
Opening with a keynote was Yevgeniya (Jane) Pinelis, chief of AI assurance for the Department of Defense (DoD) Joint Artificial Intelligence Center. Pinelis develops test and evaluation (T&E) processes that assure AI systems work as they should and that humans are trained to use them properly.
“We’ve made major progress in changing the conversation around assurance,” she said, noting a shift from the traditional view of assurance as a box to check before fielding hardware. “With the DoD moving toward being software-intensive, we’re showing AI assurance is an opportunity for an asymmetric advantage in the AI arena. Rigorous T&E helps us ensure we make more robust systems, understand performance envelopes, and use systems ethically and confidently.
Pinelis is working closely with federally funded research and development centers, including Lincoln Laboratory, on the science of AI testing. One collaboration with the Laboratory is building a “T&E Factory,” a broad set of tools to empower nonexperts in the DoD to test a model when it arrives as a black box (i.e., when the model’s inner workings are difficult to understand). “We must provide the infrastructure for nonexperts so that technologies are safe and used responsibly,” Pinelis said.
The symposium’s second keynote expanded on themes of ethical and fair AI. Tina Eliassi-Rad, a professor of computer science at Northeastern University, opened her talk with examples of discrimination or harm that can stem from biased data. She pointed to one timely example — recent studies have found that pulse oximeters, an important tool for measuring blood oxygen levels in COVID-19 patients, don’t work as well on darker skin tones. These findings have recently received national coverage, but the problem is not new; studies dating back to 1987 show this disparity.
“Imagine these data being used to train machine learning models, and they only reflect a particular subpopulation. We need researchers to be honest, acknowledging their model was trained on this subpopulation and will work only for this subpopulation,” Eliassi-Rad said.
These disparities inspired her research on using machine learning to generate “aspirational data” — data free from real-world biases. Such unbiased data, she said, are useful for recognizing and detecting other sources of unfairness in machine learning models.
To generate such data, she developed RAWLSNET, a system inspired by moral philosopher John Rawls' principles of fair equality of opportunity (FEO). According to FEO, everyone with the same talent level and willingness to use it should have the same chance of attaining employment, regardless of their socioeconomic status. RAWLSNET optimizes the parameters of a machine learning model (namely, a Bayesian network) to account for a person’s socioeconomic status and talent level when making recommendations that could, for example, help college admissions make fairer decisions.
“The synthetic data generated from ideally fair circumstances can aid policy- and decision-making,” Eliassi-Rad said.
Countering misinformation
The first session at GraphEx focused on the threat of misinformation on social media and ethical considerations when conducting research to combat this issue. “Research on countering the threat of misinformation requires rigorous methodology and experimentation to assess the effectiveness of interventions. Ethical considerations must be addressed because these experiments often involve human subjects,” said Edward Kao, the technical chair of the session.
Amna Greaves, an assistant leader in the Laboratory’s AI Software Architectures and Algorithms Group, presented research on “how to empower the American public to be more resilient to mis, dis, and mal-information.” To study this dynamic, she and her team created a rapid-play, synthetic internet simulation entitled “The Influencer.”
The purpose of the experimentation behind the serious game was to model the dynamics of misinformation and observe how automated actors (bots) could change information flows within the network. Volunteers who participated in testing were placed in one of three types of communities: pro-truthful news, pro-false news, and a mixed truthful/false news. Players were told to maximize likes within their community, and to share information pertaining to COVID-19 vaccine effectiveness. Deception was employed to obscure the fact that the community was actually composed of bots trained for a specific vaccine sentiment, and not human players. “Creating this controlled social media environment allows us to see how a person holds onto their values as they play the game,” Greaves explained.
One interesting finding from the game was evidence of the “backfire effect.” When encouraged by the bot community to share fake news, the overwhelming majority of human players worked even harder to post truthful news — in other words, “people who lean toward a certain narrative become more polarized when placed in communities of an opposite type,” Greaves said. Overall, the team found that regardless of their political bent, players were more sensitive to the presence of misinformation and better able to discern truthful information in a social media environment after playing the game. “We were encouraged by this positive outcome,” Greaves added.
Greaves also emphasized social responsibility in social media research — especially when interacting with humans, as in their Influencer game — or when using publicly available information, such as Twitter data, that users haven’t necessarily consented to being used for research.
“People are eager to get into that treasure chest and see what’s inside, but have we considered the potential risks?” she asked. This question is one of ten guiding prompts that Greaves and her team, in collaboration with Arizona State University, have developed to help AI researchers conduct socially responsible and ethical research to counter misinformation.
Advancing materials science
The second day of the symposium shifted to scientific applications of graph-based machine learning. Lin Li, a technical chair of the symposium and researcher in the Laboratory’s AI Technology Group, presented work in applying graph neural networks to expedite the discovery and design of new materials. Today, material discovery is often a long and computationally costly trial-and-error process.
“The first powered flight became operationally relevant by the development of lightweight aluminum alloys, which took 37 years. The next generation of tech, such as scalable quantum computers, will require discovery and development of novel functional materials, but we still don’t know how long it will take,” Li said.
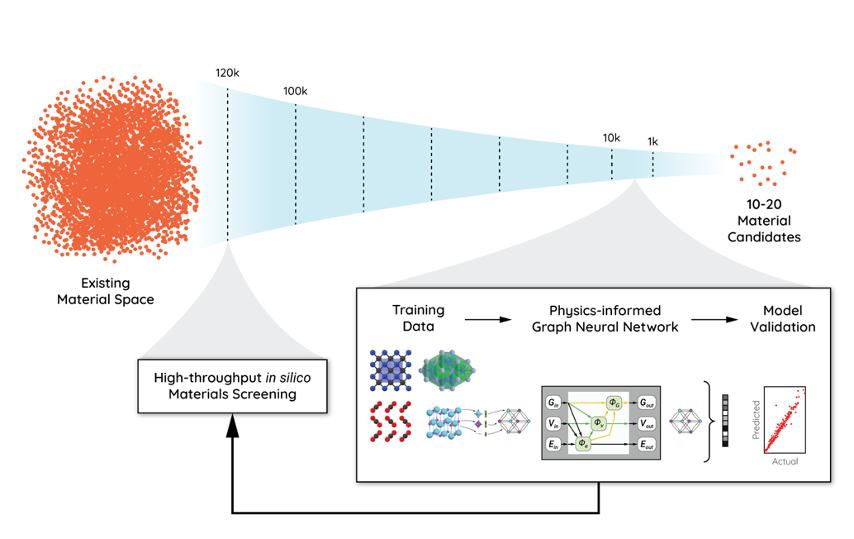
Their goal is use graph neural networks to predict materials’ thermo-mechanical properties, such as thermal conductivity, under extreme conditions, such as high temperatures. Graph neural networks combined with physics-based modeling are powerful tools for this purpose because they can learn relationships between a material’s atomic and crystalline structure and its properties under various operational conditions, and then generalize this knowledge to other materials, greatly speeding up the screening process.
“It can take weeks to identify just 10 to 20 candidate materials through physics computations,” Li said. “With machine learning, we can screen tens of thousands of materials under any temperature within minutes.”
Li’s team plans to extend this work into mission-relevant applications at the Laboratory, such as predicting electronic transport properties, which are critical to semiconductor material design.
Closing out this twelfth year of GraphEx, the symposium organizers shared their gratitude for a vibrant virtual audience, though they are eager to return to MIT’s Endicott House next year for an in-person event. “When people from different areas come together and learn from each other, it is really inspiring. I hope we all take away something worthwhile to bring back to our own applications,” said GraphEx co-chair Danelle Shah, an assistant leader in the AI Technology and Systems Group.
Technical co-chairs of the symposium were Edoardo Airoldi of Temple and Harvard Universities, and Edward Kao, Jason Matterer, and Lin Li of Lincoln Laboratory. Technical committee members included researchers from Arizona State University, Stanford University, Smith College, Duke University, the DoD, and Sandia National Laboratories. The full agenda and links to select presentations are available online.