Using machine learning to detect fake news
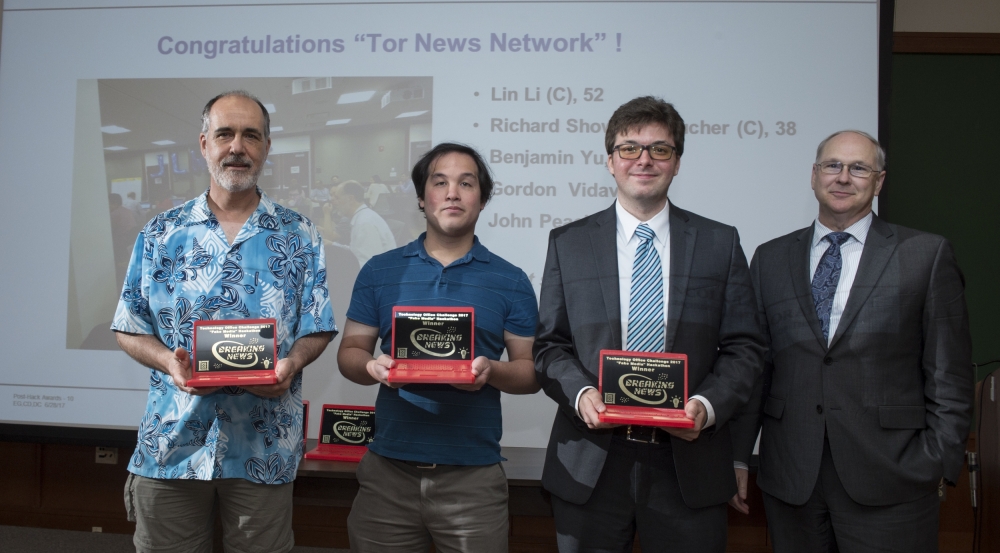
Since the 2016 presidential election, awareness of fake news has soared. Detecting and preventing the spread of unreliable media content is a difficult problem, especially given the rate at which news can spread online. With its power to erode the public's ability to make informed decisions, fake news poses a serious threat to our national security.
To combat this threat, the Lincoln Laboratory Technology Office hosted the "Fake Media" Hackathon from 8 to 9 June. The hackathon, which was the first-ever organized at the Laboratory, challenged teams of staff to use machine learning to automatically detect fake media content. The effort wrapped up with post-hack presentations on 28 June, when the three top-scoring teams and overall challenge winner were announced.
"Fake news is definitely a hot, if controversial, topic right now. Inside and outside of the Laboratory, people are very enthusiastic about doing their part to counteract the influence of false or misleading information online," said Elizabeth Godoy, a member of the Human Language Technology Group at Lincoln Laboratory. Godoy organized the hackathon with fellow group member Charlie Dagli and Deborah Campbell, Associate Technology Officer.
Forty-five participants, including staff members and interns, from across the Laboratory's divisions signed up for the challenge. The challenge organizers split participants into nine teams. During the month prior to the hackathon, teams prepared and strategized using example data—images, text, and html metadata drawn from 1600 truth-marked articles. The teams were also provided with baseline algorithms to begin developing systems. The core task of the challenge was to train systems to extract features from the data, classify the features, and fuse those classifications into a binary decision: reliable or unreliable.
"To solve the fake media challenge problem, the team must develop tools that consider all aspects of the data," said Lin Li, a staff member in the Human Language Technology Group and a captain of the winning team, Tor News Network. Teams created a variety of tools to find features in the data that could help determine if the content was fake. Tools included stance classification to determine whether a headline agreed with the article body, text processing to analyze the author's writing style, and image forensics to detect Photoshop use. Algorithms to extract even relatively simple data features, like image size, readability level, and the ratio of reactions versus shares on Facebook, proved useful in determining article reliability.
On the first day of the hackathon, the teams were given the official challenge data to put their systems to the test. Dagli led the data collection effort with the Laboratory's Open Source Data Initiative, building a dataset from annotated news websites that included more than 12,000 articles published within a two-week period in May. "It was very important for us to make sure we collected real-world data. This meant a lot of up-front work, but led to a meaningful dataset, and more importantly, realistic technical approaches," Dagli said.
Each team was allowed to submit their detection results up to five times. The organizers scored each test submission based on its rate of true detection versus false detection (i.e., the receiver operating characteristic [ROC] curve). An area under the curve (AUC) of 1.0 represents a perfect test. All teams delivered impressive results, with the top three scores clustered around .975 AUC. In between submissions, teams went to work tweaking their systems to improve their score. "A few of the handcrafted features that we introduced were surprisingly effective, improving our classifier performance by about 10%," said Cem Sahin, a top-scoring-team captain from the Cyber Analytics and Decision Systems Group.
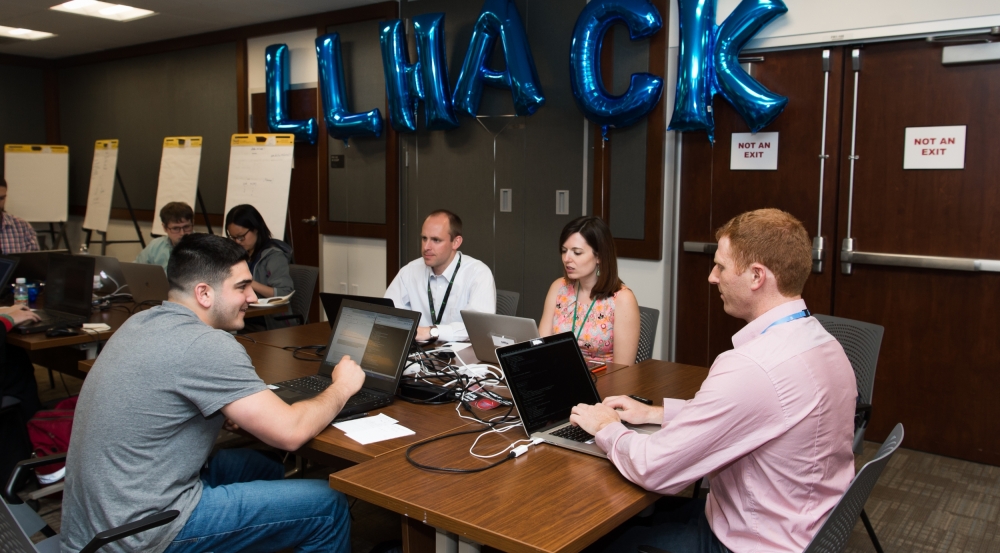
While teams aimed to outperform each other, collaboration was highly encouraged and included in the evaluation criteria used to select the challenge winner. Participants shared their tools, troubleshooting tips, and limited time with other teams during the hackathon. Staff were also encouraged to have fun, especially with team names. #SAD (Suspicious Article Detection) was voted the best name at the post-hackathon event.
During final presentations, teams shared their successes. Many expressed how much they learned in such a short period of time. "The time constraints, both during the preparation phase and during the actual hackathon, were definitely the most challenging aspects for me," said Olga Simek, a staff member from the Intelligence and Decision Technologies Group whose team placed third. "It was a great experience. Both the subject-matter experts and the team members new to the subject area learned a lot and really enjoyed the interactions with other participants."
While the challenge has concluded, it is likely that these efforts will continue. "From professors on MIT campus to industry leaders, we have been receiving a lot of requests to access our data and collaborate on different aspects of the problem," Godoy said. "Lincoln Laboratory has really jump-started technology development to combat fake news."