Green Instrumentation and Experimentation
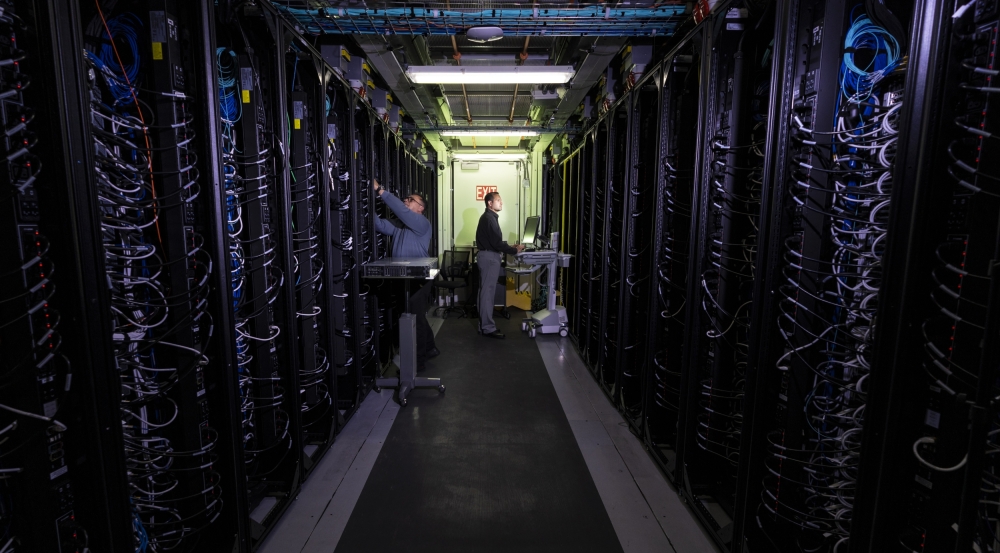
Data centers are an increasing source of carbon emissions. In particular, research, experimentation, and development of artificial intelligence (AI) can be extremely energy intensive, with deep learning being particularly demanding. Training these models carries with it a significant carbon footprint. For example, contemporary AI models such as those used in natural language processing can easily generate emissions comparable to the lifetime emission of multiple cars.
To address this growing challenge, Lincoln Laboratory is developing technologies to promote the concept of green computing, establishing foundational tools to improve efficiency, and proposing power reduction strategies. We believe these techniques will allow us to reduce the energy consumption of our Lincoln Laboratory Supercomputing Center by 20 percent. Additionally, our team is working, in collaboration with the MIT Computer Science and Artificial Intelligence Laboratory (CSAIL), to strengthen community engagement and further raise awareness on this topic; with our plans to host a Green AI Challenge, we aim to bring both the computing and AI research communities together to crowdsource, innovate, and accelerate novel solutions to address AI's carbon footprint.
Our immediate goals center on developing intelligent tools and strategies to measure and track power consumption in the data center, demonstrating the effectiveness of various power reduction strategies on AI training and experimentation. Concurrently, we are developing novel approaches aimed at reducing the computational resources required for expensive model architecture searches and exhaustive hyperparameter optimization by explicitly accounting for the energy expenditure required for model training. We plan to extend these approaches in order to cover additional computing platforms, developing power reduction strategies for diverse platforms and improving the adaptability, as well as applicability, of our technology.