Multi-label Dataset and Classifiers for Low-Altitude Disaster Imagery
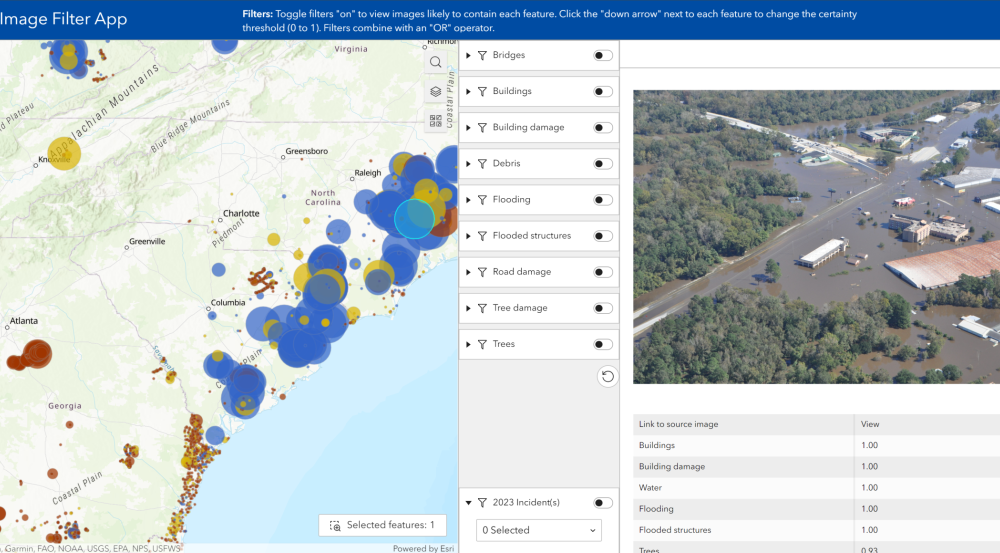
Rapid and accurate assessment of post-disaster conditions is critical for effective disaster response and recovery operations. Low altitude aerial imagery can provide valuable information about the extent and severity of damage caused by disasters. However, the quantity of these images can present a significant challenge for analysts tasked with identifying actionable information in a timely manner.
To address this challenge, Lincoln Laboratory researchers developed the Low Altitude Disaster Imagery version 2 (LADI v2) dataset and classifiers, which facilitate the development of computer vision models for identifying useful information in post-disaster aerial images. LADI v2 provides nearly 10 thousand post-disaster annotated images gathered by the Civil Air Patrol (CAP) and annotated by CAP volunteers trained in the Federal Emergency Management Agency (FEMA) damage assessment process. The annotations identify the presence of bridges, buildings, flooding, roads, trees, water, and various kinds of damage within the images. Leveraging the Lincoln Laboratory Supercomputing Center, the team used the annotated images to train computer vision classifiers to automatically label new images with these damage-related structures.
The classifiers, and the original dataset, are available on Github and Hugging Face for researchers and practitioners to use for their own purposes (a demo application is also available on ArcGIS Online). For example, the classifiers could be integrated into a data processing pipeline to automatically tag images that contain damaged buildings or flooding, allowing analysts to quickly identify images that are relevant to their assessments. The dataset and classifiers could also serve as foundations for building additional capabilities, such as supporting autonomous identification of damage from unmanned aerial systems.
For those interested in integrating this type of capability into their own system or in partnering with the Laboratory for additional research into vision-language models with automatic image captioning capabilities, contact Jeffrey Liu.