Intelligent Tornado Prediction Engine
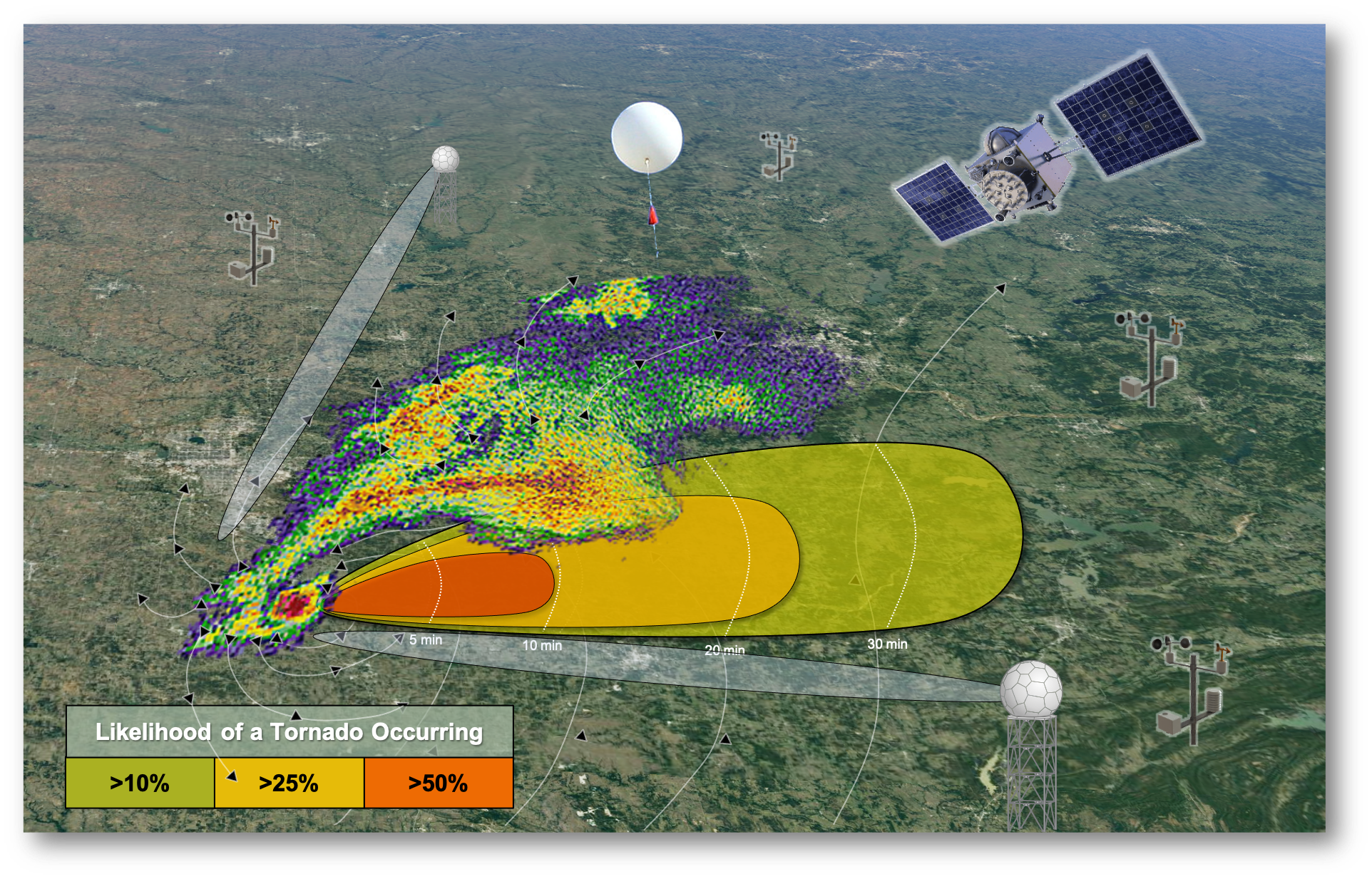
About 1,200 tornadoes occur in the United States each year, causing loss of life, thousands of injuries, and billions of dollars in economic impact. Tornadoes are notoriously difficult to forecast, resulting in high false-alarm rates and short lead-times for warnings. New paradigms must be explored for predicting these events, using the plethora of data now available with the advent of rapid-update satellite, radar, and numerical weather prediction models.
One of the most rapidly advancing approaches to many problems in the atmospheric sciences is a form of artificial intelligence (AI) called deep learning. Deep learning models are capable of combining many large datasets to “learn” trends and features in the data based on history. When a large number of tornadic cases are combined with a large number of null cases (from similar-looking storms), deep learning can potentially discern between tornadic and non-tornadic precursors.
To train and validate such deep learning models, we compiled a massive open-source dataset called TorNet. Containing more than 200,000 radar images, TorNet is intended as a benchmark to advance tornado research within the machine learning and weather science communities.
Using TorNet, we developed tornado detection models. Our deep learning model performed similar or better than all others known in the literature, correctly classifying over 85% of tornadoes rated EF-2 or higher. We also evaluated other types of models to compare against. The parameters of these models are freely available and described in our paper.
Additionally, we developed tornado prediction models that operate at 5-, 10-, and 15-minute lead times. These models are trained on TorNet images depicting a storm prior to tornadogenesis, allowing the models to learn precursors at different amounts of time leading up to a tornado forming. The models could help guide forecasters' attention to storm cells most likely to produce tornadoes. We also used these models to explore explainable AI techniques, which provide reasoning for the models' output in understandable terms that align with a forecaster's criteria for issuing a warning.
Overall, these models could form the basis for enhanced decision support systems, which hold potential for increasing warning lead times and lowering false-alarm rates. Such improvements could allow for more effective public response and lower the risks to populations in the path of these events.